What are the two main current challenges of marine insurance, according to Meetrisk, an insurtech company which rates marine risks?
Firstly, an adverse combined ratio, and secondly the difficulty to leverage the abundant and accessible data available, despite data being the raw material of the underwriting business.
In this context, we believe that the key to data science is to address the heart of the insurance value chain, by improving:
- risk knowledge, with data science segmenting risk profile, identifying weak signals, rating and predicting the risk,
- the productivity and expense of quickly providing underwriters with more accurate risk knowledge,
- matching pricing with each specific risk profile.
The Meetrisk Risk rating leverages and correlates both technical data (vessels, safety, risk management, etc.) and behavioural factors (managerial and organisational) of shipping companies to precisely identify each marine risk profile. Such a rating consequently provides a homogeneous and objective view of the risk, supporting the underwriting business: risk selection, insurance pricing and capital allocation.
Beyond the underwriting business, this rating supports the sustainability of the marine business by identifying and predicting risk trends allowing for more precise loss prevention activities for the benefit of the whole system (insured clients, brokers, insurers, reinsurers).
Lastly, our opinion, as an insurtech, regarding the hurdles in achieving such innovation projects are:
- lack of ambition due to heavy data legacy,
- lack of awareness regarding cleaning and structuring data: we know that data science projects are 80% dedicated to data preparation and about 20% for the data science itself,
- difficulty to make innovation and insurance experts work together, leading to build several heavy data tools without immediate return of investment (ROI) for insurer teams,
- difficulty to explain the outputs of the algorithms.
To address these hurdles there needs to be:
- a strong alliance between the business and data science teams with a good balance between ambition and pragmatism,
- and agility in providing quick ROI for underwriting teams,
to ensure data science success for marine insurance and its profitability.
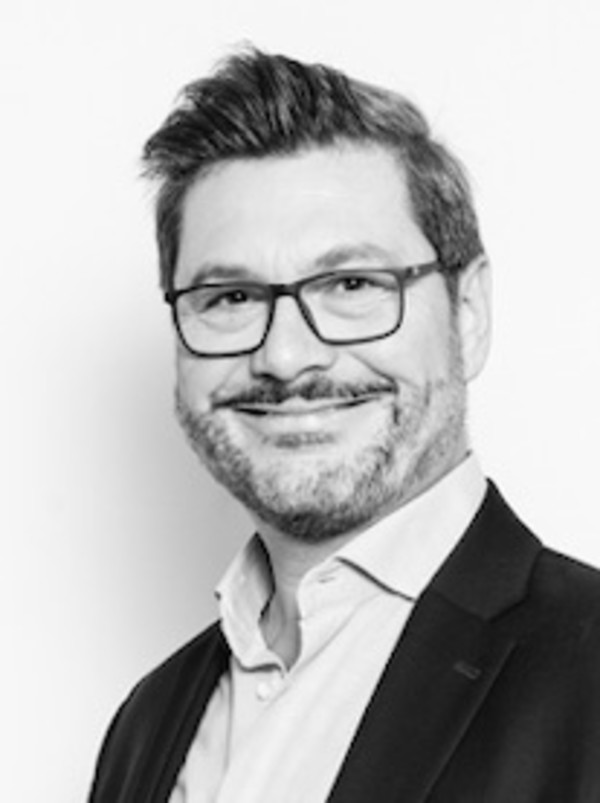